10-step AI enablement model
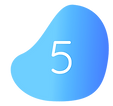
5 | Tech & Data Readiness
ASSESSING INFRASTRUCTURE, DATA QUALITY AND INTEGRATIONS FOR AI IMPLEMENTATION
AI is only as effective as the data it processes. Poor data quality or incompatible systems can derail AI projects before they even start, and add unforeseen costs into your business case.
Ensuring high-quality data is foundational for AI success. However, many organisations struggle with data that is inconsistent, siloed, or unstructured, leading to unreliable AI models. In short - crap in, crap out. With trust being one the biggest blockers to employee adoption of AI tools, its important that data is is fixed upfront as one too many inaccurate results will result in a total loss of confidence.
As with all technology implementations the new shiny toys need to play nicely with the older ones. Existing infrastructure may not be equipped to handle the demands of AI workloads, necessitating significant upgrades in data storage, processing capabilities, and network architectures, and for AI to create real value it needs to seamlessly integrate with systems and processes, requiring engagement with process owners and impacted system owners
Experimenting with AI models and evaluating the outputs will be critical to understanding how your data is performing, and significant investment could be required to bring data up to scratch to ensure output is accurate and trustworthy.
To view the detailed step-by-step guide to Tech & Data Readiness and get access to full library of FFF Knowledge Articles and templates please get in touch.